Domain-Aware Continual Zero-Shot Learning
- Kai Yi
- Mohamed Elhoseiny King Abdullah University of Science and Technology (KAUST), Saudi Arabia
Abstract
We introduce Domain Aware Continual Zero-Shot Learning (DACZSL), the task of visually recognizing images of unseen categories in unseen domains sequentially. We created DACZSL on top of the DomainNet dataset by dividing it into a sequence of tasks, where classes are incrementally provided on seen domains during training and evaluation is conducted on unseen domains for both seen and unseen classes. We also proposed a novel Domain-Invariant CZSL Network (DIN), which outperforms state-of-the-art baseline models that we adapted to DACZSL setting. We adopt a structure-based approach to alleviate forgetting knowledge from previous tasks with a small per-task private network in addition to a global shared network. To encourage the private network to capture the domain and task-specific representation, we train our model with a novel adversarial knowledge disentanglement setting to make our global network task-invariant and domain-invariant over all the tasks. Our method also learns a class-wise learnable prompt to obtain better class-level text representation, which is used to represent side information to enable zero-shot prediction of future unseen classes.
DACZSL Setting
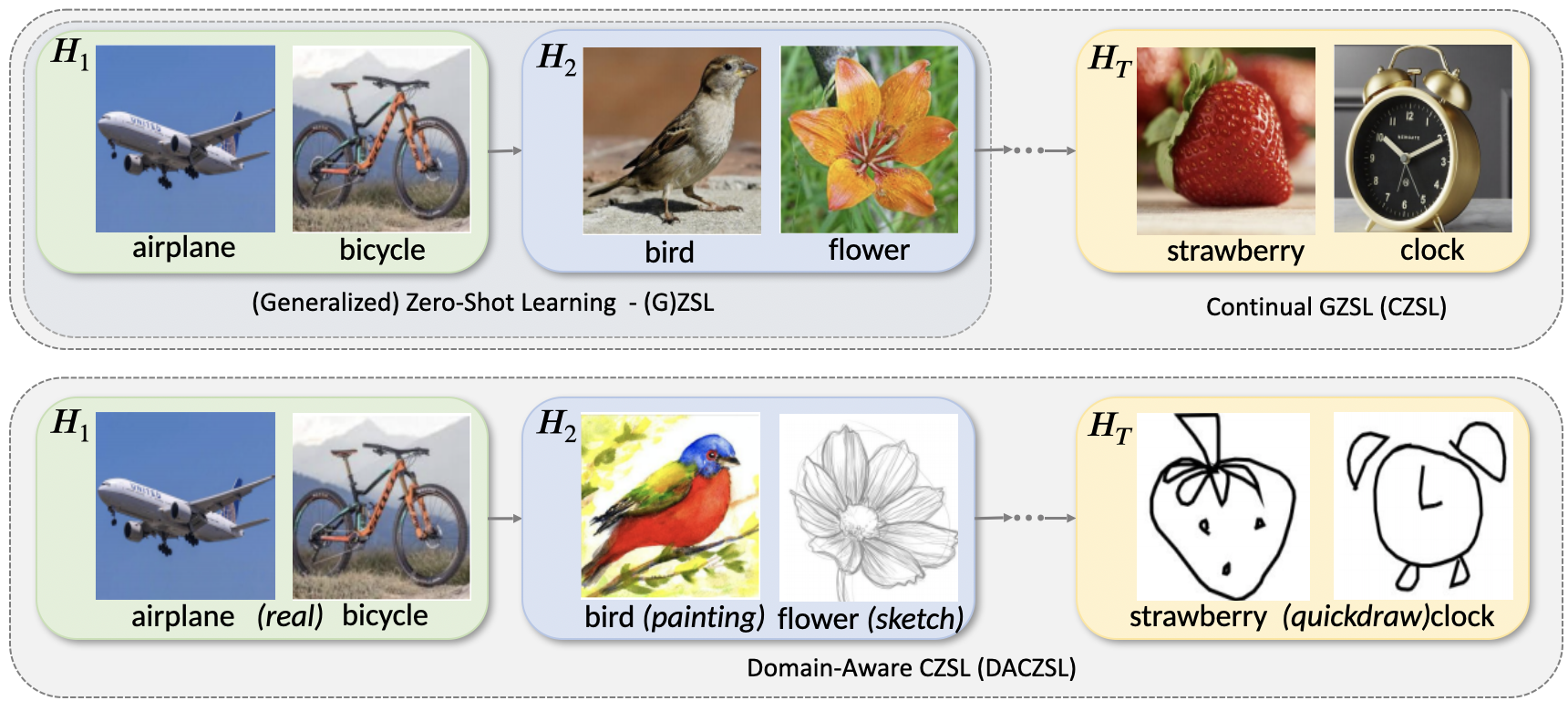
Method: Domain-Invariant Continual Zero-Shot Learning Network (DIN)
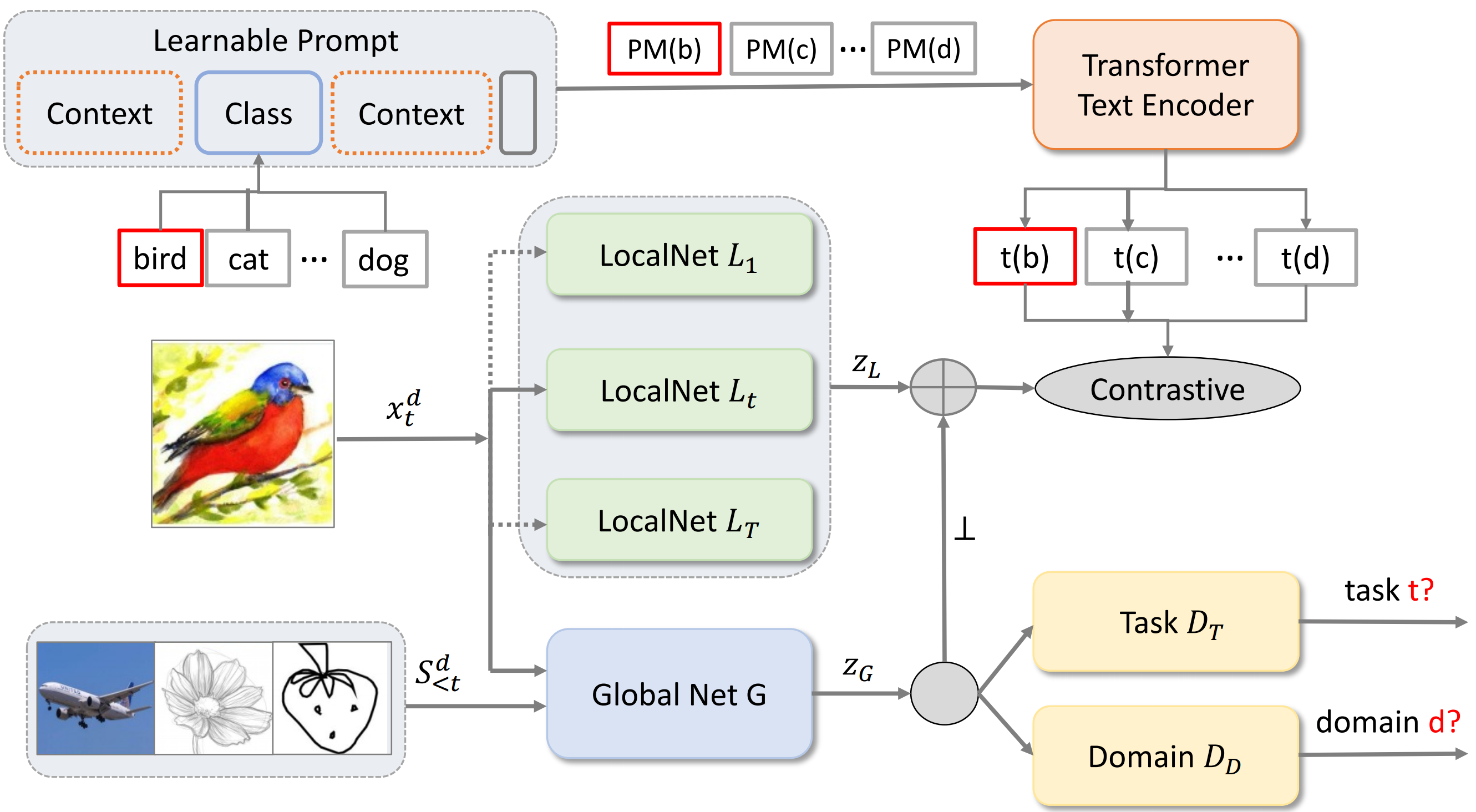
Generalized Domain-Aware Zero-Shot Learning (GDAZSL) Results
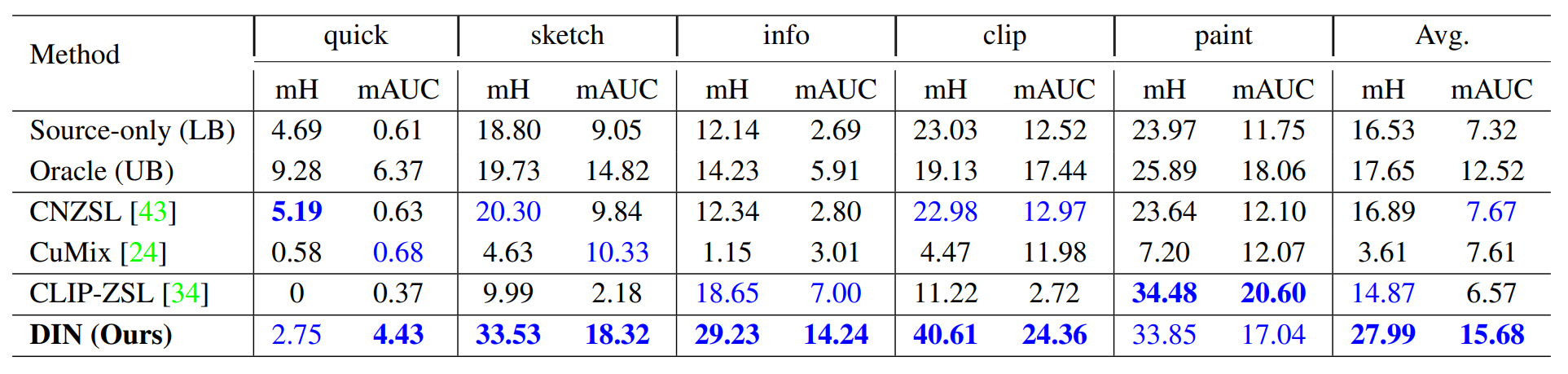
Domain-Aware Continual Zero-Shot Learning Results

Citation
If you find our work useful in your research, please consider citing:@article{yi2021domain, title={Domain-Aware Continual Zero-Shot Learning}, author={Yi, Kai and Elhoseiny, Mohamed}, journal={arXiv preprint arXiv:2112.12989}, year={2021} }